βββββββββββββββββββββββββββββββββββββββββββββ βββββββββββββββββββββββββββββββββββββββββββββββββββββββββββββββββββββββββββββ
ββββββββββββββββββββββββββββββββββββββββββββββ ββββββββββββββββββββ βββββββββββββββββββββββββββββββββ ββββββββββββββ
ββββββββββββββββββββββββββββββββββββββββββββββ βββββββββββββββββββ βββββββ ββββββββββββββββββββ ββββββββββββββ
ββββββββββββββββββββββββββββββββββββββββββββββ ββββββββββββββββββββββββββββββββββββββββββββββββββββββββββββββ βββββββββββββ
βββββββ ββββββββββ βββββββββββββββββββββββββββ ββββββββββ βββββββ βββββββββββββββββββββββββββββββββ ββββββββββββββ
βββββββ ββββββββββ βββββββββββββββββββββββββββ ββββββββββ βββββββ βββββββββββββββββββββββββββββββββ ββββββββββββββ
βββββββ ββββββββ βββββββββββββββββββββββββββ ββββββββ βββββββββββββββββββββββββββββββββββββββββββββββββββββββββββββββββ
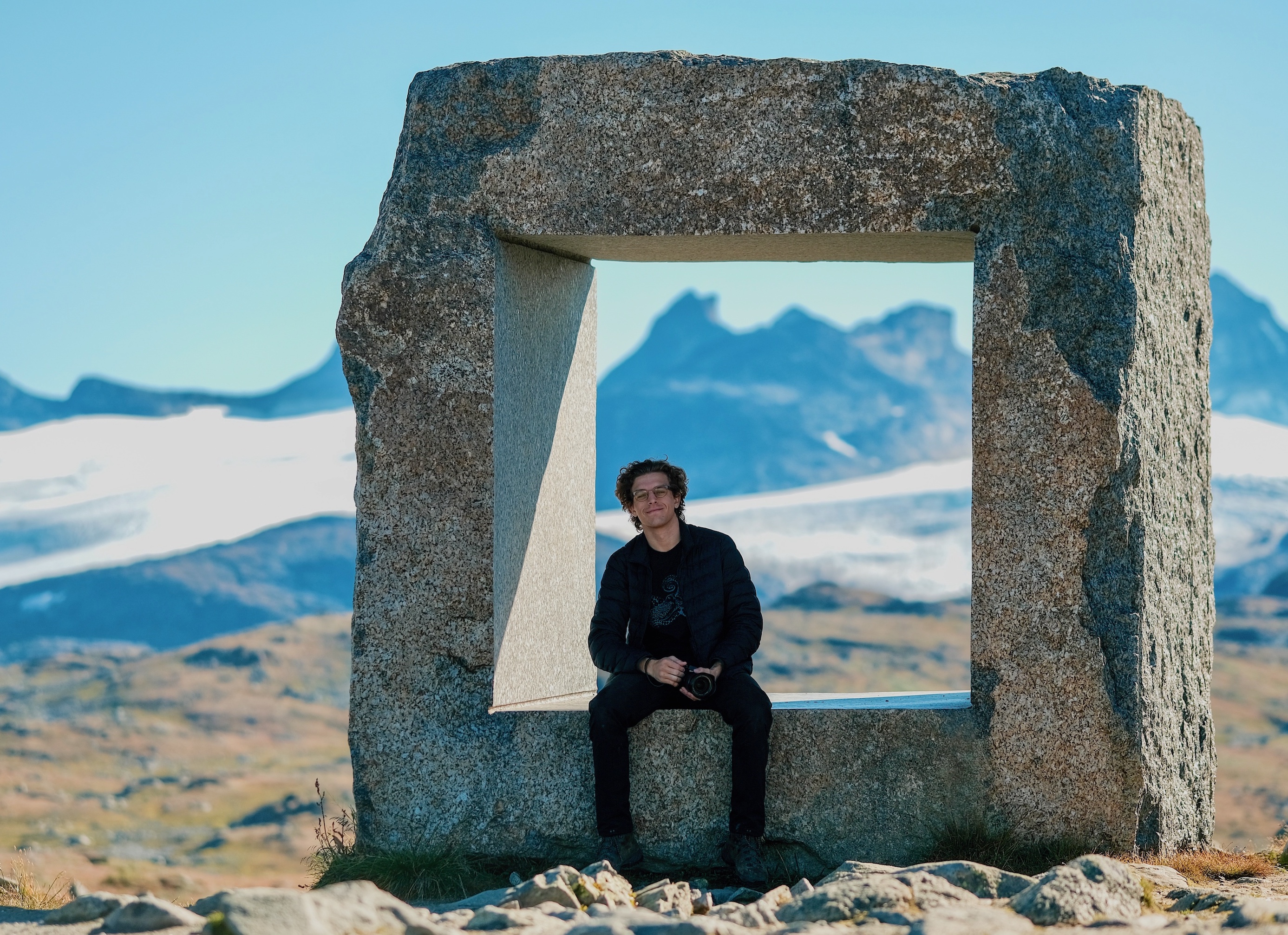
Hi, Iβm Ivan.
Iβm a humanitarian-turned-techie, born in St. Petersburg, Russia, and currently undertaking a PhD in Edinburgh, Scotland. I care a lot about artificial intelligence and its impacts on the world, and Iβm working on making these impacts beneficial.
In my spare time, I enjoy reading anything I can get my hands on (send me recommendations!), working on personal projects (send me ideas, too!), photography, and occasional pyrography and woodcarving. I read a lot, and I hope to make writing on this blog a regular exercise, because it helps me think.
My interests
- Artificial intelligence
- Machine learning
- Cognitive science
- Entrepreneurship
- Political theory
- Philosophy
- Economics
- Photography
I will probably write about all of these on this blog at one point or another.
Work
I am currently undertaking a PhD at the University of Edinburgh as part of the Center for Doctoral Training in NLP.
Research
Broadly speaking, I work on enabling human-like generalization in AI systems β I believe that the ability to learn from few-shot examples and broadly generalize out-of-domain not just to new inputs, but to new tasks and goals, is a fundamental prerequisite for general intelligence. Open to collaboration - if you are interested, please contact me.
Contact Me
Iβm reachable on my Twitter/X, and you can also email me at <myfirstname> @ <thisdomain>
with just about anything!